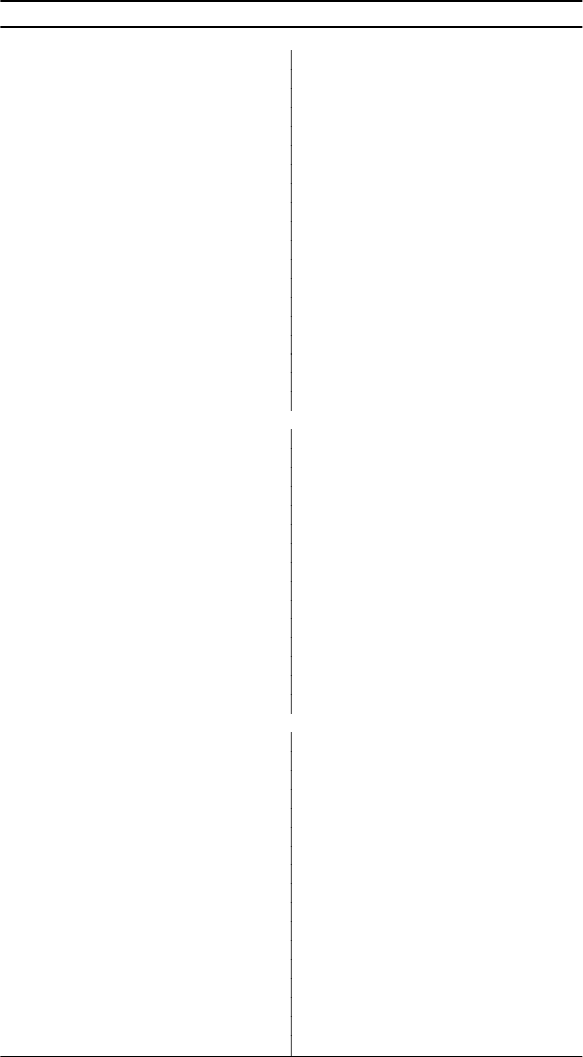
Table 3 (continued)
Yea r Age Pred. Act. Resid. Year Age Pred. Act. Resid.
Mark McGwire Paul Molitor
1987 24 0.981 0.987 0.007 1978 22 0.805 0.673 -0.132
1988 25 0.991 0.830 -0.161 1979 23 0.822 0.842 0.020
1989 26 0.997 0.806 -0.191 1980 24 0.835 0.809 -0.025
1990 27 1.001 0.859 -0.142 1982 26 0.851 0.816 -0.035
1991 28 1.002 0.714 -0.288
1983 27 0.855 0.743 -0.112
1992 29 1.001 0.970 -0.031 1985 29 0.855 0.764 -0.091
1995 32 0.992 1.125 0.134 1986 30 0.853 0.765 -0.087
1996 33 0.987 1.198 0.211 1987 31 0.850 1.003 0.153
1997 34 0.981 1.039 0.058 1988
32 0.846 0.836 -0.010
1998 35 0.974 1.222 0.249 1989
33 0.841 0.818 -0.023
1999 36 0.966 1.120 0.155 1990
34 0.835 0.807 -0.028
1991 35 0.828 0.888 0.060
1992 36 0.820 0.851 0.031
1993 37 0.811 0.911 0.101
1994 38 0.801 0.927 0.127
1995 39 0.790 0.772 -0.017
1996 40 0.778 0.858 0.081
1997 41 0.764 0.786 0.022
1998 42 0.750 0.718 -0.033
Sammy Sosa B.J. Surhoff
1990 22 0.854 0.687 -0.167
1987 23 0.732 0.773 0.041
1991 23 0.870 0.576 -0.294
1988 24 0.745 0.611 -0.134
1993 25 0.893 0.794 -0.099
1989 25 0.755 0.626 -0.129
1994 26 0.900 0.884 -0.016
1990 26 0.762 0.706 -0.056
1995 27 0.904 0.840 -0.063
1991 27 0.766 0.691 -0.075
1996 28 0.904 0.888 -0.016
1992 28 0.766 0.635 -0.131
1997 29 0.903 0.779 -0.124 1993 29 0.765 0.709 -0.056
1998 30 0.901 1.024 0.122 1995
31 0.760 0.870 0.109
1999 31 0.898 1.002 0.103 1996
32 0.756 0.834 0.078
2000 32 0.894 1.040 0.145 1997
33 0.751 0.803 0.052
2001 33 0.889 1.174 0.285 1998
34 0.745 0.789 0.044
2002 34 0.883 0.993 0.110 1999
35 0.738 0.839 0.101
2003 35 0.876 0.911 0.035 2000 36 0.730 0.787 0.057
2004 36 0.868 0.849 -0.020 2001 37 0.721 0.726 0.004
2004 40 0.688 0.785 0.097
Larry Walker Rafael Palmeiro
1990 24 0.967 0.761 -0.207
1988 24 0.893 0.785 -0.108
1991 25 0.977 0.807 -0.170 1989 25 0.903 0.728 -0.175
1992 26 0.984 0.859 -0.125 1990 26 0.910 0.829 -0.081
1993 27 0.988 0.841 -0.147 1991 27 0.914 0.922 0.008
1994 28 0.988 0.981 -0.007 1992 28 0.914 0.786 -0.128
1995 29 0.987 0.988 0.001 1993 29 0.913 0.926 0.013
1997 31 0.982 1.172 0.189 1994 30 0.911 0.942 0.031
1998 32 0.978 1.075 0.096 1995 31 0.908 0.963 0.055
1999 33 0.974 1.168 0.195 1996 32 0.904 0.927 0.023
2001 35 0.961 1.111 0.151 1997 33 0.899 0.815 -0.085
2002 36 0.952 1.023 0.071 1998 34 0.893 0.945 0.051
2003 37 0.943 0.898 -0.046 1999 35 0.886 1.050 0.164
2000 36 0.878 0.954 0.076
2001 37 0.869 0.944 0.075
2002 38 0.859 0.962 0.103
2003 39 0.848 0.867 0.019
2004 40 0.836 0.796 -0.040
• Act. = actual OPS, Pred. = predicted OPS, Resid. = Act. - Pred.
• Resid. sums to zero across time for each player.
• Valu e s of Resid. greater than one standard error are in bold.
•
Equation is OPS line 1 in Table 1. Standard error is .0757.
• Resid. in 2001 for Palmeiro is .0750.
16
Journal of Quantitative Analysis in Sports, Vol. 4 [2008], Iss. 1, Art. 1
DOI: 10.2202/1559-0410.1074
Brought to you by | Yale University Library New Haven (Yale University Library New Haven)
Authenticated | 172.16.1.226
Download Date | 3/28/12 11:34 PM